by Lee Gesmer | Apr 26, 2024 | Noncompete Agreements
I’ve written about noncompete law what feels like countless times on this blog. The most important post was the one in 2018 announcing that the Massachusetts legislature had placed so many restrictions and conditions on noncompetes that they were, for all practical purposes, obsolete in the state. See A New Era In Massachusetts Noncompete Law.
Now the Federal Trade Commission has taken steps to outlaw noncompetes nationally. Claire MacCollum at Gesmer Updegrove LLP has written a client advisory summarizing the FTC’s actions. Click on the image below to read it.

by Lee Gesmer | Feb 4, 2024 | General
In October 2023 a Missouri jury awarded class-action plaintiffs $1.8 billion in a federal antitrust suit against the National Association of Realtors (NAR) and several brokerage firms. As I discuss below, the central issue in this case – and what I expect will be the central issue on appeal – is whether the case should have been tried under the “per se” rule or the “rule of reason.” Spoiler: while the NAR may ultimately be found liable for violating the antitrust laws the trial judge erred in trying the case as a “per se” violation. I expect the Eighth Circuit or the Supreme Court to reverse the judgment in this case.
Per Se or Rule of Reason?
A key issue in any Sherman Act Section 1 case is whether the challenged conduct should be tried under the per se rule or the rule of reason. Some anticompetitive conduct is viewed as so presumptively harmful that it’s treated as a “per se” violation, meaning that no offsetting pro-competitive justification defense is allowed. Classic examples are horizontal price fixing, market division and bid rigging. In a civil case these violations can result in money damages. In a criminal case the corporate employees involved may be facing time in a federal prison.
Per se antitrust conspiracies usually occur in secret. The classic scenario is the secret meeting where competitors agree to fix prices or divide markets. But this is not always the case. Sometimes the challenged conduct takes place in the open. That’s the situation in Burnett v. NAR (W.D. Miss.), the case that resulted in the $1.8 billion verdict against the NAR. (docket).
The NAR Mandatory Payment Rule
If you’ve ever sold a house in the U.S. it’s likely you hired a realtor to represent you. This person is referred to as the “seller-broker” or the “listing-broker.” You likely knew that a second realtor might be involved – the “buyer-broker” – and that both brokers would receive a commission on sale.
When you retained your broker you agreed to pay a brokerage commission when the house was sold. It was probably between 5% and 6% of the sale price, although you might have
negotiated a lower amount. You may also have been aware that this commission would be split 50-50 between the two brokers. You, the seller, would be paying both brokers from the proceeds, a fact that probably was a consideration when you set the selling price for the house. If you expected to sell your house for $1 million, a 5% brokerage fee meant that at closing you’d pay $25,000 to each broker.
Perhaps you told your realtor that while you were OK paying him 2 ½% on sale, you didn’t want to pay the buyer-broker. After all, the buyer-broker represented the buyer – let the buyer negotiate its own brokerage fee and pay it directly. You may have pointed out that many buyer-side brokers play a minimal role in home purchasers today, when buyers can research the housing market online. A buyer-broker’s job might be not much more than providing access to a lock box and communicating an offer. Why, you ask, should the buyer’s broker be paid $25,000, an amount that may be unrelated to the service provided?
If that’s what you were thinking you may have instructed your broker to post your house for sale with “buyer-broker compensation to be determined.”
If you tried this your broker likely responded that deferring the buyer-broker’s commission until later in the transaction was not an option. Why? Because your broker is almost certainly a member of the NAR, and must comply with the NAR membership rules. Not stating the buyer-broker’s commission up front would run afoul of a long-standing NAR rule that the seller must state the buyer-broker commission on MLS when the property is first listed. If the seller didn’t agree to this the listing-broker would lose access to the NAR-owned MLS. And, your broker adds, even in the age of Zillow and Redfin an MLS listing is essential to advertising a house and finding a buyer.
If a realtor told you this they would be right. Since the mid-1990s the NAR’s so-called “Mandatory Payment Rule” (the “NAR Rule”) has required that any seller listing on MLS must make an offer to potential buyer brokers when the property is first listed:
In filing property with the multiple listing service, participants make blanket unilateral offers of compensation to the other MLS participants and shall therefore specify on each listing filed with the service the compensation being offered by the listing broker to the other MLS participants. (NAR Handbook, Section 5)
In plain English, this rule (along with others not quoted here) requires that a seller broker disclose an offer of compensation to the buyer broker when the house is first listed, despite not having any information about the buyer, the buyer-broker or the services the buyer-broker will perform. Any negotiation of the compensation must occur before the property is shown and cannot be negotiated after that point. As a practical matter, therefore, there rarely if ever is a negotiation over the compensation offered to buyer brokers on the MLS.
The NAR Case
The NAR Rule has been in existence since the mid-1990s, and it has long been the target of criticism and controversy. This finally came to a head when several home sellers filed an
antitrust class action in Missouri against the NAR and several large brokerage firms. The NAR and some large realtors refused to settle or back down, and at trial things didn’t go well for the NAR and its broker co-defendants. A jury found that the policy violated the antitrust laws and awarded $1.8 billion in damages. Add to this the possibility of treble damages exceeding $5 billion and attorneys fees. And this is just the beginning of a litigation free-for-all – the verdict has led to similar lawsuits in other jurisdictions and increased government scrutiny of the NAR.
The Per Se Rule – When Does It Apply?
The per se rule is a draconian rule in the context of antitrust law, and the Supreme Court has been clear that it is to be applied cautiously. In fact, in recent decades the Court has been liberal in recharacterizing per se conduct as rule of reason conduct.
The leading case on this is Leegin v. PSKS (U.S. 2007), where the Court moved resale price maintenance from the “per se” column to the “rule of reason” column. However, the Supreme Court has done this more than once. In Continental v. Sylvania (U.S. 1977), non-price vertical restrictions were moved into the rule of reason column.
Leegin, Sylvania and similar cases have important implications for the “per se” vs “rule of reason” decision. In these decisions the Supreme Court has made clear that the per se rule should be applied only after the courts have adequate experience with the restraint at issue. See also Arizona v. Maricopa County Medical Society (U.S. 1982)(“Only if it is clear from the record that the agreement is so plainly anticompetitive that no elaborate study of its effects is needed to establish its illegality may a court properly make a per se judgment”).
The Court emphasized this in Leegin:
The accepted standard for testing whether a practice restrains trade in violation of § 1 of the Sherman Act is the rule of reason, which requires the factfinder to weigh “all of the circumstances,” including “specific information about the relevant business” and “the restraint’s history, nature, and effect.” The rule distinguishes between restraints with anticompetitive effects that are harmful to the consumer and those with procompetitive effects that are in the consumer’s best interest. However, when a restraint is deemed “unlawful per se,” the need to study an individual restraint’s reasonableness in light of real market forces is eliminated. Resort to per se rules is confined to restraints “that would always or almost always tend to restrict competition and decrease output.” Thus, a per se rule is appropriate only after courts have had considerable experience with the type of restraint at issue, and only if they can predict with confidence that the restraint would be invalidated in all or almost all instances under the rule of reason. [citations omitted; emphasis added]
In order to obtain the “considerable experience” required make a determination of this sort courts typically receive evidence in the form of lay testimony and expert opinion (from economists and industry experts), review the economic literature and reach a reasoned determination whether the practice at issue “always or almost always” restricts competition and decreases output.
Is The NAR Policy A Per Se Antitrust Case?
In the NAR case the trial judge decided the all-important “per se” vs. “rule of reason” issue at the summary judgment stage: “The Court agrees with Plaintiffs and finds that the per se rule is applicable here . . . The record creates a genuine material fact as to whether Defendants have engaged in a horizontal price-fixing scheme, exactly the situation where applying the per se rule is appropriate.” (Emphasis added). By the time of trial the judge had concluded that there was no longer an issue of material fact on this issue – in his view the case fell under the per se rule, and he instructed the jury accordingly.
Consequently, the NAR defendants went to trial with one hand tied behind their backs – they were not allowed to argue the economic benefits of the NAR Rule. They were limited to defending against the allegations of a conspiracy and the damages claims.
However, if you reread the NAR Rule that I quoted above you will notice something unusual about it – it does not “fix” the commissions paid to the listing and buyer’s broker. In other words, it doesn’t say that the brokers will charge (and split) a 5% or 6% commission – the listing broker can set whatever commission he agreed upon with his client, the seller. While listing brokers must make an offer of compensation, the amount of the offer is unrestricted. The commission offered could – at least in theory – be as little as $1. And, the Rule does not affect the amount of the selling broker’s commission – sellers are free to negotiate that amount with their brokers.
In other words, although the judge characterized this Rule as a “horizontal price-fixing scheme,” the NAR Rule does not “fix” prices – it only requires that a non-zero offer must be made, and when.
The NAR has a strong argument that the courts do not have sufficient experience with a rule of this nature in the residential real estate market, and therefore placing this practice under the per se rule was legal error by the trial judge. If this argument prevails the verdict and any injunction imposed by the district court as part of the final judgment (which has yet to be entered) will be reversed by either the Eighth Circuit or the Supreme Court. The plaintiffs would then have the option of retrying the case under the rule of reason.
The Verdict is In, But the Case is Far From Over
In the meantime, it’s important to bear in mind that this case is still in early innings. The parties are only now filing post-trial briefs, and the defendants are asking the trial court to set aside or reduce the verdict. The plaintiffs will ask the judge to issue an injunction prohibiting the parties from following the NAR Rule, and if he doesn’t reverse the jury verdict he likely will do so, although the precise terms of an injunction are uncertain.
If the verdict does hold up on appeal what impact will this case have on the U.S. residential real estate market? Will the NAR lose its ownership and control over the MLS? Will home buyers have the option of paying their brokers directly, and if so will this lower the overall cost of home purchases? Or is the “seller pays both brokers” business model so deeply entrenched in the real estate industry that it will continue on its own momentum, without an NAR Rule to compel it?
It’s also worth noting that the NAR faces significant legal challenges in addition to this suit, not the least of which is a wide ranging Department of Justice antitrust investigation that was pending settlement under the Trump administration, but which has been reopened under the Biden Department of Justice.
The future is always uncertain, but all of these legal issues and uncertainties add up to a challenging future for the real estate brokerage industry.
by Lee Gesmer | Nov 27, 2023 | Copyright, General
If you follow developments in artificial intelligence, two recent items may have caught your attention. The first is a Copyright Office submission by the VC firm Andreessen Horowitz warning that billions of dollars in AI investments could be worth less if companies developing this technology are forced to pay for their use of copyrighted data. “The bottom line is this . . . imposing the cost of actual or potential copyright liability on the creators of AI models will either kill or significantly hamper their development.”
The second item is OpenAI’s announcement that it would roll out a “Copyright Shield,” a program that will provide legal defense and cost-reimbursement for its business customers who face copyright infringement claims. OpenAI is following the trend set by other AI providers, like Microsoft and Adobe, who are promising to indemnify their customers who may fear copyright lawsuits from their use of generative AI.
Underlying these two news stories is the fact that the explosion of generative AI has the copyright community transfixed and the AI industry apprehensive. The issue is this: Does copyright fair use allow AI providers to ingest copyright-protected works, without authorization or compensation, to develop large language models, the data sets that are at the heart of generative artificial intelligence? Multiple lawsuits have been filed by content owners raising exactly this issue.
The Technology
The current breed of generative AIs is powered by large language models (LLMs), also known as Foundation Models. Examples of these systems are ChatGPT, DALL·E-3, MidJourney and Stable Diffusion.
This technology requires that developers collect enormous databases known as “training sets.” This almost always requires copying millions
of images, videos, audio and text-based works, many of which are protected by copyright law. When the data is scraped from the web this is potentially a massive infringement of copyright. The risk for AI companies is that, depending on the content (text, images, music, movies), this could violate the exclusive rights of reproduction, distribution, public performance, and the right to create derivative works.
However, for purposes of copyright fair use analysis it’s important to recognize that the downloads are only an intermediate step in creating an LLM. Greatly simplified, here’s how it works:
In the process of creating an LLM model words are broken down into tokens, numerical representations of the word. Each token is a unique numerical ID. The numerical IDs are then transformed into high-dimensional vectors. These vectors are learned during the model’s training and capture semantic meanings and relationships.
Through multiple layers of transformation and abstraction the LLM identifies patterns and correlations within the data. Cutting edge systems like GPT-4 have trillions of parameters. Importantly, these are not copies or replications of the copyright-protected input data. This process of transformation minimizes the risk that any output will be infringing. A judge or jury viewing the data in an LLM would see no similarity between the original copyrighted text and the LLM.
Is Generative AI “Transformative“?
Because the initial downloads in this process are copies, they are technically a copyright infringement – a “reproduction.” Therefore, it’s up to the AI companies to present a legal defense that justifies the copying, and the AI development community has made it clear that this defense is based on copyright fair use. At the heart of the AI industry’s fair use argument is the assertion that AI training models are “non-expressive uses.” Copyright protects expression. Non-expressive use is the use of copyrighted material in a way that does not involve the expression of the original material.
For the reasons discussed above, the AI industry has a strong argument that a properly constructed LLM is a non-expressive use of the copied data.
However, depending on the specific technology this may be an oversimplification. Not all AI systems are the same. They may use different data sets. Some, but not all, are designed to minimize “memorization” which makes it easier for end users to retrieve blocks of text or infringing images. Some systems use output filters to prevent end users from utilizing the LLM to create infringing content.
For any given AI system the fair use defense turns on whether the LLM is trained and filtered in such a way that its outputs do not resemble protected inputs. If users can obtain the original content, the fair use defense is more difficult to sustain.
There is a widespread assumption in the AI industry that, assuming an AI is designed with adequate safety measures, using copyright-protected content to train LLMs is shielded by the fair use doctrine. After all, the reasoning goes, the Second Circuit allowed Google to create a searchable index of copyrighted books under fair use. (Google Books, Hathitrust). And the Supreme Court permitted Google to copy Oracle’s Java API computer code for a different use. (Oracle v. Google). AI companies also point to cases holding that search engines, intermediate copying for the purpose of reverse engineering and plagiarism-detection software are transformative and therefore allowed under fair use. (Perfect 10 v. Google; Sega Enterprises v. Accolade; A.V. et al. v. iParadigms)
In each of these cases the use was found to be “transformative.” So long as the act of copying did not communicate the original protected expression to end users it did not interfere with the original expression that copyright is designed to protect. The AI industry contends that LLM-based systems that are properly designed fall squarely under this line of cases.
How Does Generative AI Impact Content Owners?
In evaluating AI’s fair use defense the commercial impact on content owners is also important. This is particularly true under the Supreme Court’s decision earlier this year in Warhol Foundation v. Goldsmith. In Warhol the Court taught that, in a case that involved commercial copying of photographs, the fact that the copies were used in competition with the originals weighed against fair use.
AI developers will argue that, so long as users can’t use their generative AI systems to access protected works, there is no commercial impact on content owners. In other words, like in Google Books, the AI does not substitute for or compete with content owners’ original protected expression. No one can use a properly constructed AI to read a James Patterson novel or listen to a Joni Mitchell song.
The AIs should be able to distinguish Warhol by pointing out that they are not selling the actual copyrighted books or images in their data sets, and therefore – like in Google Books – they are causing the content owners no commercial harm. In other words, the AI developers will argue that the “intermediate copying” involved in creating and training an LLM is transformative where the resulting model does not substitute for any author’s original expression and the model targets a different economic market.
Does the authority of Google Books and the other intermediate copying cases extend to the type of machine learning that underpins
generative AI? While the law regulating AI is in its infancy, several recent district court cases have given plaintiffs an unfriendly reception. In Thomson Reuters v. Ross Intelligence the defendant used West’s head notes and key number system to train a specialized natural language AI for lawyers. West claimed infringement. A Delaware federal district court judge denied Ross’s motion for summary judgment based on fair use, and held that the case must be decided by a jury. However, relying on the intermediate copying cases, the judge noted that Ross would have engaged in transformative fair use if its AI merely studied language patterns in the Westlaw headnotes and did not replicate the headnotes themselves. Since this is in fact how LLMs are trained on data, Ross’s fair use defense likely will succeed.
In a second case, Kadrey v. Meta, the plaintiffs, book authors, claimed that Meta’s inclusion of their books in its AI training data violated their exclusive ownership rights in derivative works. The Northern District of California federal judge dismissed this claim. The judge noted that the LLM models could not be viewed as recasting or adapting the plaintiff’s books. And, the plaintiffs had failed to allege that the content of any output was infringing. “The plaintiffs need to allege and ultimately to prove that the AI’s outputs incorporate in some form a portion of the plaintiffs’ books.” Another N.D. Cal. case, Andersen v. Stability AI is consistent with these rulings.
While these cases are early in the evolution of the law of artificial intelligence they suggest how AI developers can take precautions to insulate themselves from copyright liability. And, as discussed below, the industry is already taking steps in this direction.
The Industry Is Adapting To The Copyright Threat
In the face of legal uncertainty, the AI industry is adapting to legal risks. The potential damages for copyright infringement are massive, and the unofficial Silicon Valley motto – “move fast and break things” – doesn’t apply with the stakes this high.
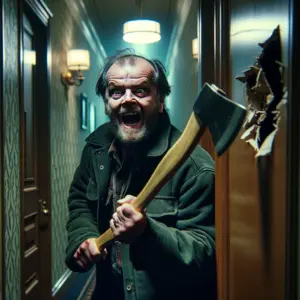
ChatGPT4: Create an image showing Jack Nicholson in The Shining
Early in the current generative AI boom (only a year ago) it was possible to use some of these systems to generate copyright- protected content. However, the dominant AI companies seem to have plugged this hole. Today, if I ask OpenAI’s ChatGPT to provide the lyrics to “All Too Well” by Taylor Swift it declines to do so. When I ask for the text of the opening paragraph of Stephen King’s “The Shining,” again it refuses and tells me that it’s protected by copyright. When I ask OpenAI’s text-to-image creator Dall·E for an image of Batman, Dall·E refuses, and warns me that what it will create will be sufficiently different from the comic book character to avoid copyright infringement.
These technical filters are illustrative of the ways that the industry can address the copyright challenge, short of years of litigation in the federal courts.
The first, and most obvious, is to train the systems not to provide infringing output. As noted, Open AI is doing exactly this. The Shining may have been downloaded and used to create and train Chat GPT, but it won’t let me retrieve the text of even a small part of that novel.
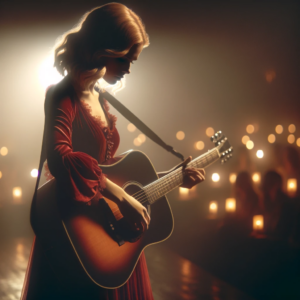
ChatGPT4: Create an image of Taylor Swift performing her song “All Too Well”
Another technical measure is minimization of duplicates of the same work. Studies have found that the more duplicates that are downloaded and processed in an LLM the easier it is for end-users to retrieve verbatim protected content. “Deduplication” is a solution to this problem.
Another option is to license copyrighted content and pay its creators. While this would be logistically challenging, a challenge of similar complexity has been met in the music industry, which has complex licensing rules that address different types of music licensing and a centralized database system to make that process accessible. If the courts prove to be hostile to AI’s fair use defense the generative AI field could evolve into a licensing regime similar to that of music.
Another solution is for the industry to create “clean” databases, where there is no risk of copyright infringement. The material in the database will have been properly licensed or will be comprised of public domain materials. An example would be an LLM trained on Project Gutenberg, Wikipedia and government websites and documents.
Given the speed at which AI is advancing I expect a variety of yet-to-be conceived or discovered infringement mitigation strategies to evolve, perhaps even invented by artificial intelligence.
International Issues
Copyright laws vary significantly across countries. It’s worth noting that there has been more legislative activity on the topics discussed in this
post in the EU than the US. That said, as of the date of this post near the close of 2023 there is no consensus on how LLMs should be treated under EU copyright law.
Under a recent proposal made in connection with the proposed EU “AI Act,” providers of LLMs would need to “prepare and make publicly available a sufficiently detailed summary of the content used to train the model or system and information on the provider’s internal policy for managing copyright-related aspects.”
Additionally, they would need to demonstrate “that adequate measures have been taken to ensure the training of the model or system is carried out in compliance with Union law on copyright and related rights . . .”
The second of these two provisions would allow rights holders to opt out of allowing their works to be used for LLM training.
In contrast, the recent US AI Executive Order orders the Copyright Office to conduct a study that would include “the treatment of copyrighted works in AI training,” but does not propose any changes to US copyright law or regulations. However, US AI companies will have to pay close attention to laws enacted in the EU (or elsewhere), since – as has been the case with the EU’s privacy laws (GDPR) – they have the potential to become a de facto minimal standard for legal compliance worldwide.
Andreessen Horowitz and the Copyright Shield
What about the two news items that I mentioned at the beginning of this post? With respect to the Andreessen Horowitz warning of the cost of copyright risk on AI developers, in my view the risk is overstated. If AI developers design their systems with the proper precautions, it seems likely that the courts will find them to qualify for fair use.
As to OpenAI’s promise to indemnify end users, the risk to OpenAI is slim, since its output is rarely similar to inputs in its training data and its filters are designed to frustrate users who try to output copyrighted content. In any event end users are rarely the targets of infringement suits, as seen in the many copyright suits that have been filed to date, which all target only AI companies as defendants.
The Future
The application of US copyright law to LLM-based AI systems is a complex topic. I expect more lawsuits to be filed as what appears to be a massive revolution in artificial intelligence continues at breakneck speed. While traditional copyright law seems to favor a fair use defense, the devil is in the details of these complex systems, and the legal outcome is by no means certain.
***
Selected pending cases:
Andersen v. Stability AI, N.D. Cal.
J.L. v. Alphabet Inc., N.D. Cal.
P.M. v. OpenAI, N. Dist. Cal.
Doe v. GitHub, N.D. Cal
Thomson Reuters Enter. Ctr. GmbH v. Ross Intel. Inc., D. Del.
Kadrey v. Meta, N.D. Cal.
Sancton v. OpenAI, S.D. N.Y.
Doe v. GitHub, N.D. Cal.
by Lee Gesmer | Nov 21, 2023 | Corporate Law, Employment
OpenAI fired Sam Altman, its CEO-founder, the afternoon of November 17, 2023. In the corporate chaos that followed the company went through two acting CEOs within three days. By the end of the day on Monday, November 20, Altman and other key executives at OpenAI were hired by Microsoft. Immediately following this 700 employees – practically the whole company – signed a letter threatening to quit unless the Board resigns and Altman is reinstated.
It’s hard to imagine how this termination could have been handled more ineffectively. It is an exemplar of exactly how NOT to fire your CEO.
However, all the OpenAI Board members had to do was ask their own product – ChatGPT – how to go about terminating Altman. Here’s my query to ChatGPT-4, and it’s response –
PROMPT TO GPT:
I am on the Board of Directors of a closely held California-based corporation. We are considering terminating our CEO. Please tell me in
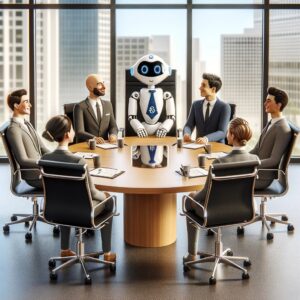
To provide better governance and ensure rational decision-making, GPT-5 will join the new OpenAI Board of Directors
detail what steps we should take to prepare for and execute this termination. Take into consideration that my company is very high profile – we are a cutting-edge technology company that receives a lot of attention from the press and the technology industry. Our CEO is one of the founders of the company, and he is effectively “the face” of the company. He is frequently interviewed by the industry press and often appears at industry conferences and events to talk about our products. We have many partnerships with other companies and the CEO has been instrumental in forming those partnerships. While we are a bit unsure about this, we think that many of our key employees respect the CEO and may be loyal to him
CHATGPT-4 RESPONSE (my comments in italics and [brackets]):
Given the high-profile nature of your company and the CEO’s prominent role as a founder and the face of the company, the process of terminating the CEO requires additional layers of consideration and caution. Here are detailed steps tailored to your situation:
Preliminary Confidential Discussions: Initiate confidential discussions among the Board of Directors to thoroughly assess the reasons for considering termination and the potential impact on the company. Given the CEO’s prominence, it’s essential to evaluate the risks and benefits of this action carefully. [OpenAI board appears not to have done this]
Legal Consultation: Engage legal counsel experienced in high-profile executive terminations and corporate law. They can provide advice on contractual obligations, potential legal risks, and strategies for minimizing legal exposure, especially if the CEO is also a shareholder or has a significant stake in the company. [No information on whether Altman has an employment agreement with OpenAI or what its terms are. Note, however, that noncompete restrictions are not enforceable under California law, and therefore Altman and the other OpenAI employees cannot be prevented from going to work at Microsoft for this reason. There may, however, be trade secret issues in this fact pattern
Review Employment Agreement and Corporate Bylaws: Scrutinize the CEO’s employment contract and the corporation’s bylaws. These
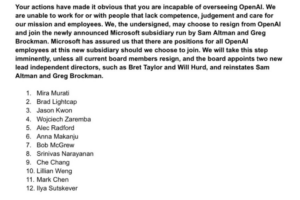
Employee Letter to OpenAI Board
documents will guide the legal and procedural aspects of the termination process, including any ‘for cause’ conditions, severance terms, and required board approvals. Conduct a risk assessment focusing on potential repercussions within the technology industry, impact on partnerships, employee morale, and public perception. This assessment should consider the possibility of the CEO’s departure affecting key relationships and projects. [The OpenAI board either failed to do this, or completely miscalculated]
Internal Alignment: Ensure alignment and consensus within the Board of Directors. Given the CEO’s foundational role in the company, board members must be unified in their decision and prepared for the subsequent steps. [Ilya Sutskever, one of the Board members that voted to terminate Altman on Nov. 17, signed the employee letter on Monday Nov. 20]
Plan for Succession and Transition: Develop a succession plan that includes identifying an interim CEO or a successor. This plan should address how to maintain continuity in leadership and reassure employees, partners, and stakeholders about the company’s stability and future direction. [OpenAI announced an interim CEO on Friday – Mira Murati – and on Monday replaced her with a person brought in from outside the company. Over a period of four days OpenAI had three CEOs]
Communications Strategy: Formulate a comprehensive communications strategy. This should include internal communication to
employees, as well as external communication to the press, industry partners, and stakeholders. The message should be crafted to maintain the company’s reputation and market confidence. [Internal/external communications were almost nonexistent]
Managing Employee Sentiment: Prepare for potential fallout among employees who may be loyal to the CEO. This could involve individual discussions with key team members, departmental meetings, and ensuring that HR is equipped to handle any concerns or unrest. [Seems not to have been done at all]
Board Approval and Documentation: Formalize the decision with a board vote and document the process meticulously. This documentation is crucial for legal compliance and for maintaining a record of the decision-making process. [Outside of the board, no one seems to know why Altman was fired]
Executing the Termination: Plan the termination meeting with the CEO carefully, considering the timing, location, and who will be present. The conversation should be handled with respect and sensitivity, acknowledging the CEO’s contributions to the company. [Termination was done via Google Meet, a video service similar to Zoom]
Public Announcement: Coordinate the public announcement of the CEO’s departure with the communications strategy. This announcement should be respectful and align with the narrative agreed upon by the board. [The board didn’t have a narrative]
Post-Termination Steps: Monitor the company’s internal and external environment after the termination. Be prepared to manage any unforeseen consequences, including media inquiries, market reactions, and internal dynamics.
After the termination, review the company’s policies, leadership structure, and strategic direction. This is an opportunity to learn from the experience and strengthen the company’s governance and leadership practices.
Throughout this process, the key is to balance legal and procedural diligence with sensitivity to the human and cultural aspects of this significant change. The termination of a high-profile CEO can have far-reaching implications, and managing it effectively requires a thoughtful, strategic approach.
– end of GPT response –
by Lee Gesmer | Oct 26, 2023 | Copyright, General
By Lee Gesmer and Andrew Updegrove
Every citizen is presumed to know the law … and it needs no argument to show… that all should have free access to its contents.
U.S. Supreme Court in Georgia v. Public.Resource.Org (2020)
Many private organizations promulgate best-practice standards. Two examples you might be familiar with are the National Fire Protection Association (NFPA) and the American Society for Testing and Materials (ASTM).
In the U.S., unlike most foreign countries, standards are developed “from the bottom up” by the private sector, rather than “from the top down” by government agencies or quasi-public bodies. In keeping with this division of labor, government agencies have come to rely extensively on private sector standards developers to provide standards suitable for adoption as regulations.
Federal law permits federal agencies to incorporate privately developed standards into law by referencing them in the Federal Register without reproducing them there. The Code of Federal Regulations (CFR) has more than 27,000 incorporated standards. States and municipalities do this as well, adding to that number. Once adopted the standards carry the force of law.
These private-government relationships are crucially important – they leverage specialized knowledge and industry expertise to formulate robust and reliable standards that the government could not create by itself, and save untold millions of tax dollars in avoided costs for government agencies that would otherwise have to generate them. The standards organizations that provide standards referenced into law, in turn, gain legal legitimacy and wider application for their standards.
There is also a commercial side to these relationships – many standards organizations support themselves in part by the sale of their standards.
Public.Resource.Org (Public Resource) is a nonprofit group that disseminates legal materials. Its website has posted thousands of standards, including those produced and copyrighted by ASTM. ASTM (along with two other standards organizations) sued Public Resource for copyright infringement. The case has been working its way through the courts for a decade. Absent a successful appeal to the Supreme Court, the Court of Appeals for the District of Columbia finally decided the issue on September 12, 2023. It held that the non-commercial dissemination of these standards as incorporated by reference into law constitutes copyright fair use, and therefore cannot support liability for copyright infringement. 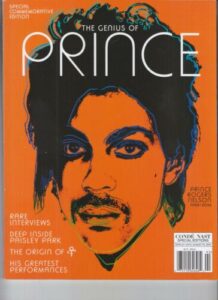
As we have observed on many occasions, copyright fair use is an unpredictable legal doctrine. Often, the outcome seems to be in the eye of the beholder – the judge or judicial panel – rather than the result of any predictive legal test. A recent example of this is Goldsmith v. Warhol: a federal district court held that Warhol’s use of Goldsmith’s photo of Prince was fair use. The Second Circuit reversed, holding that it wasn’t. The Supreme Court upheld that ruling, but under a different rationale from the Second Circuit. Three courts, three different approaches to fair use. For another example see Final Thoughts On Google v. Oracle. The result is a confusing body of law that lacks predictability for the copyright community, both authors and the lawyers that are asked to advise them.
D.C. Circuit’s Holding in ASTM
While Warhol involved art and Oracle software, ASTM involved privately developed technical standards that had been incorporated into law “by reference.”
There is no question that in most cases technical standards are copyrightable – that is, they reflect sufficient originality to be protected by U.S. copyright law. Hence, without an affirmative defense Public Resource’s reproduction and distribution of ASTM’s standards infringed its copyrights. Public Resource’s defense was copyright fair use.
The D.C. Circuit applied – as it must – the four-factor fair use test:
Purpose and Character of the Use. Under the first factor it found that the “purpose and character“ of Public Resource’s nonprofit status favored fair use. Further Public Resource’s use of the standards – to provide a free repository of the law – is “transformative,” a key issue in any fair use case. While in most cases the term “transformative” involves changes to the work, here the court construed it to mean a transformative “use” of the work.
Nature of the Copyrighted Work. Factor two also favored fair use. Because the court viewed the standards as “factual” in nature – a conclusion we find questionable – it conclude that they “fall at best, at the outer edge of copyright’s protective purposes.” Factual works are often given “weak” or “thin” copyright protection, and because protection is weaker for such works, it’s easier to establish fair use.
Amount and Substantiality of the Portion Used. Under factor three, although Public Resource copied the standards in their entirety, the court found that this was necessary in light of the purpose. “If an agency has given legal effect to an entire standard, then its entire reproduction is reasonable in relation to the purpose of the copying . . ..” This is not unusual in the context of copyright fair use – many fair use cases involve comprehensive copying. Oracle is a good example of this.
Effect of the Use Upon the Potential Market for or Value of the Copyrighted Work. Lastly, the fourth fair use factor required the court to consider the “market harm“ caused by Public Resource’s copying, including any substantially adverse impact on the “potential market“ for the original standards. While the court observed that it “seems reasonable” to suppose that economic harm might result, it found that the plaintiffs could not quantify past or future financial harms, relying instead on “conclusion, assertions and speculation.“ In any event, even if Public Resource’s free postings lowered the demand for the plaintiffs’ standards, this was outweighed by “the substantial public benefits of free and easy access to the law.“ The court concluded that the fourth fair use factor did not tip the balance one way or the other. But because the first three factors “strongly“ favored fair use, it found that Public Resource’s non-commercial posting of standards incorporated into law by reference is fair use.
Legal Precedents Favored Public Resource
The extent to which the law should be in the public domain is not a new issue for copyright law. In 2020 the Supreme Court held that annotations to Georgia’s official statutory code, as government edicts, were free from copyright. In that case the Court didn’t even reach fair use – it held that officials who “speak with the force of law” cannot claim copyright in the works they create in the course of their official duties.” Georgia v. Public Resource.
The lower courts have also weighed in on this issue. In Veeck v. Southern Building Code the Fifth Circuit relied on fair use to hold that model building codes adopted by reference could be copied. In Building Officials & Code Administration. v. Code Technologies, Inc. the First Circuit suggested that once a model building code has been adopted into law it is in the public domain, and remanded for further consideration.
Public Resource relied heavily on these cases on appeal, and indeed, these precedents put ASTM and its co-plaintiffs in an uphill battle heading into the appeal to the D.C. Circuit.
Copyright Fair Use Based On “Public Benefits”
While not explicitly identified in the Copyright Act, the “public benefit” theory of fair use prioritizes societal and cultural benefits in the application of copyright law. A recent example of this is the Supreme Court’s holding in Oracle v. Google. In this 2021 case the issue was whether Google’s use of Oracle’s Java API (Application Programming Interface) in its Android operating system constituted fair use. While Google copied all of Oracle API and used it commercially, the Court found fair use, based in part on the benefit to the software development industry and technical innovation. As the Court said, “we must take into account the public benefits the copying will likely produce.”
Similarly, in the 2015 Google Book Search decision, Author’s Guild v. Google, the Second Circuit recognized the substantial public benefits of Google’s project in concluding that Google’s verbatim copy of books was protected by fair use.
The D.C. Circuit’s ruling in the ASTM case follows this line of reasoning. Just as there is a public benefit in allowing software developers to use the Java API, and a public benefit in allowing the public to search copyright-protected books for relevant “snippets,” so does the publication of laws incorporated by reference benefit the public by making the law more accessible. However, as we discuss below, it did this at the risk of upsetting the delicate balance between the standards organizations and the governments that benefit from their works.
Was the “Public Benefits” Theory of Fair Use Properly Applied in ASTM?
While the D.C. Circuit’s holding allowing the unauthorized reproduction of standards may fall within the “public benefits” line of fair use cases, in our view there is a risk that the court misjudged the interplay between standards organizations, government entities, and public access. Any challenge to the delicate symbiotic private-government relationship risks injury to the public interest, which benefits from the creation of these standards. Based on our experience working with nonprofit standards organizations for decades, we fear that the D.C. Circuit underestimated this potential disruption.
Importantly, the court found insufficient an accommodation that many standards developers (including ASTM) have already put in place in response to Public Resource’s challenge. Specifically, they have created public “reading rooms” where every standard they have developed that has been incorporated into law by reference can be read, free of charge, online in read-only mode. The American National Standards Institute (ANSI) hosts an “IBR Standards Portal” offering one stop access to the incorporated by reference (IBR) standards of a dozen major standards organizations can be accessed, as well as links to another sixteen standards organizations reading rooms with links to their own IBR’d standards.
As noted, many standards organizations charge a fee for copies of their standards. In the case of many traditional standards developers, such fees comprise a major, or even the majority, of the budgets of the organizations. Developing standards is inherently time-consuming and expensive, and in some cases (e.g., organizations that develop building codes), most or all of the production of such organizations is referenced into law. In other cases, standards were never intended for referencing into law, but have been nonetheless, without notice to, or consent by, the organization that developed them. The revenues from sales and licensing are reinvested into research, development, and enhancement of new and existing standards. Respecting copyright protects the investments of these organizations in developing standards, ensuring they can fund their continuing operations and standards development and providing incentives to continue to create these essential public goods.
The unauthorized distribution by nonprofits risks reducing those revenues and incentives by offering a free alternative to purchasing or licensing the standards. This, in turn, risks slowing down the frequency of updating existing standards and innovating new ones, potentially leaving them outdated or less applicable to evolving industry needs.
This may prove to be the case if the implications of the decision extend beyond nonprofit vendors to for-profit companies. Some for-profit companies already do sell copies of standards without first paying for the rights to do so. It is difficult to see how the court’s rationale – finding fair use when a nonprofit engages in this behavior – does not extend to for-profit sales of standards.
Our bottom line takeaway: the implications of this decision on private standard-setting organizations and their business models may be far reaching. Hopefully, there may be a legislative solution that may provide relief. On March 17, 2023, Darrell Issa (R CA) introduced proposed amendments to amend the Copyright Act with bipartisan support from seven representatives from each party. If enacted, the bill would void a fair use defense against a claim of infringement of an IBR’d standard if that standard “is displayed for review in a readily accessible manner on a public website,” without cost.
We support this common sense ratification of the public reading room approach and hope that the bill is adopted.
American Society for Testing and Materials v. Public.Resource.Org., Inc. (D.C. Cir. Sept. 12, 2023).